False discovery rate.
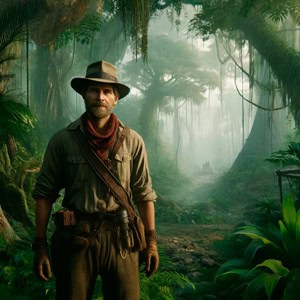
When multiple hypothesis tests are performed, the probability of committing a type 1 error increases, increasing the risk of detecting false positive effects. The false discovery rate allows to limit the probability of type 1 error when the number of contrasts is very high, also allowing to control the risk of making type 2 errors and failing to detect true positives.
Few of you will know Percival Harrison Fawcett, Percy to his friends. And although he is unknown to the general public, the same is not true of the character that was created inspired by his story. Someone named Indiana Jones.
Percy was an English explorer who, in the early 19th century, embarked on a series of expeditions to the heart of the Amazon in search of a legendary city known as the Lost City of Z, which he believed to be El Dorado, the legendary city that It was supposed to exist in the unexplored jungle of Brazil.
Armed with ancient maps, indigenous stories, and his fierce determination, Fawcett and his team crossed rivers, jungles, and faced numerous dangers. However, the Amazon rainforest was full of “decoys”: pottery shards, remains of abandoned villages, and natural formations that often looked like clues to the Lost City. Each time Fawcett encountered one of these possible clues, his heart filled with hope, only to face disappointment when the evidence turned out to be a false lead.
We do not know if Fawcett ever discovered the Lost City, since he disappeared in the jungle with his son Jack and a friend of his son, Raleigh Rimmell, on May 29, 1925, but we can be sure of his persistence in following every clue, immune to fever and fatigue, despite the numerous false positives and deceptions with which the jungle tried to prevent its objective.
To me, this attitude seems similar to the challenge that scientists face with multiple comparisons in their research, especially in those fields in which big data and databases with thousands of variables are rampant, such as in modern “omics” sciences.
In this statistical jungle, each analysis may seem like a promising lead, but without adequate methods to control errors, researchers can end up following many false leads. If Fawcett had a method to better discern which clues were worth pursuing, he could have optimized his search and perhaps found his lost city.
In a similar way, researchers use the false discovery rate to balance the risk of false positives and maximize the probability of significant findings. Keep reading this post and we will see how we can keep false positives at bay, always inevitable and almost obligatory when numerous multiple comparisons are made.
The formulation of the problem
We already saw in a previous post how the type 1 error is one of our enemies to defeat on the battlefield of research.
When we make a comparison between two groups, we propose a hypothesis test in which we establish a null hypothesis that, in general, says the opposite of what we want to prove. In most cases it will be assumed, under the null hypothesis, that the observed differences are due solely to chance.
In this way, we calculate the value of p, which is nothing more than finding a difference like the one found or greater due to chance, with the null hypothesis being true. We usually set the threshold of 0.05, so that if p < 0.05 we consider it unlikely that the difference is due to chance and we reject the null hypothesis: with this we “demonstrate” that the difference is due to the intervention or exposure in study.
You see that I have written “demonstrate” in quotes, and this is because we can never be sure of making the correct decision, since there is a probability of 5% (0.05) that the null hypothesis that we are rejecting as false is, actually, true. We will be detecting an effect that does not exist, a false positive. This is type 1 error.
Think about it for a moment, in 1 out of every 20 hypothesis tests in which the null hypothesis is rejected we screw up and detect a false positive.
Well, this figure, which may seem small to some, grows when we carry out various contrasts, as we saw in a previous post.
When we perform multiple comparisons in the same study, the probability of type 1 error (the so-called alpha) in each individual contrast is 0.05, but the overall alpha increases with the number of comparisons. We can calculate the global alpha, which is the probability of obtaining at least one false positive, as 1-0.95n, with n being the number of comparisons.
If we do a hypothesis test, the probability of obtaining a false positive is 0.05 (5%). But if we perform 10 contrasts in the same study, this probability rises to 0.4 (40%): if we repeat the study many times, in almost 1 in 2 we will commit at least one false positive.
Imagine in a genomic association study, in which the association of a certain trait implies, for example, 100 genes studied. In this case, the probability of obtaining at least one false positive is 0.99 (99%). If this seems like a lot, consider that these types of studies usually make thousands or tens of thousands of comparisons.
There is no doubt, we must control false positives.
The simple but insufficient solution
We already saw that there are several methods to adjust the p threshold to consider each contrast significant and keep the global alpha below 0.05. In these cases, no matter how many comparisons we make, the overall probability of obtaining at least one false positive remains below 0.05.
The simplest method and one of the most used is the Bonferroni method, which consists of dividing the usual value of p (which is usually 0.05) by the number of contrasts or comparisons, thus obtaining the new threshold of statistical significance for each contrast.
In the example of the 10 contrasts, the new p value would be 0.05 / 10 = 0.005. This means that, to reject the null hypothesis in each individual contrast, we have to obtain a value of p < 0.005.
On a global level this method seems a good solution. If we calculate the probability of obtaining at least one false positive with the previous formula (substituting 0.95 for 0.995), we obtain a value of 1 – 0.99510 = 0.0488. We see that the global alpha remains below the conventionally chosen value of 0.05.
Now let’s think about what’s wrong with this method. The p value to reject the null hypothesis is 10 times lower. This means that the probability of committing a type 2 error will increase: not reaching statistical significance and not being able to reject the null hypothesis when, actually, it is false. Simply put, it increases the risk of not detecting effects that actually exist and falsely attributing the difference to chance.
If this happens with 10 comparisons, imagine the example of the 100 genes. Here, the new p = 0.05 / 100 = 0.0005. It will be increasingly difficult to detect true positives with such a low p value. We will have managed to keep false positives at bay, but at a too high price: not being able to detect true positives.
This is where a different way of approaching the topic comes into play: the false discovery rate.
False discovery rate
We have already seen that the Bonferroni method is too restrictive when the number of comparisons is high.
We know that, when we make many comparisons, among all the contrasts in which we reject the null hypothesis there will be a variable number of false positives.
In this way, we can consider a method that ensures that the proportion of false positives does not exceed a certain value with respect to the number of total rejections, be it 10%, 20% or whatever value we consider most convenient.
The so-called false discovery rate (FDR) is thus defined as the expectation of the proportion of false discoveries (incorrect rejections of the null hypothesis) over the total number of discoveries (rejections of null hypotheses).
If we call 𝑉 the number of false positives and 𝑅 the total number of rejections, this proportion (actually not a rate) can be expressed according to the following formula:
FDR = E(V / R)
E represents the expected mean for this proportion. What does expected mean? Let’s imagine that we repeat the study countless times. If we set a FDR of 20%, we will obtain, on average, 20% false positives. In some studies there will be more and in others there will be less. Random, our inseparable companion.
This approach, useful when a high number of comparisons are made, allows greater flexibility and statistical power in the detection of true effects, avoiding the extreme conservatism of other methods such as Bonferroni’s. In essence, FDR allows researchers to manage the balance between discovering real effects and minimizing false positives more efficiently and pragmatically.
Let’s see how we can set the new p value to keep the FDR at the value we choose since, unlike the p value, there is no general agreed value and it will depend on the data and the context of the study.
The Benjamini–Hochberg method
The Benjamini-Hochberg method is one of the simple alternatives for calculating the p value that allows us to control the desired FDR. To do this, this algorithm follows a series of sequential steps:
1. Specify the maximum value of false discoveries that we want when performing multiple comparisons. Let’s call this value that controls the FDR, q.
2. Calculate the p values for the m hypothesis tests that we want to perform.
3. Order the m p values from lowest to highest.
4. We select, from among the values of p, those that are less than the result of multiplying q by the order number of p and dividing it by the total number of contrasts (m).
5. This value will be the new statistical significance value. The p value of a given contrast must be lower to reject the null hypothesis.
In case it is not understood well, let’s see a simple example. I am going to put only 5 comparisons, although we already know that, in practice, many more are usually made.
Suppose the p values of the 5 contrasts are 0.001, 0.583, 0.123, 0.012, and 0.473. We want an FDR = 10% (0.1).
First, we sorted the p values from lowest to highest: p1 = 0.001, p2 = 0.012, p3 = 0.123, p4 = 0.473, and p5 = 0.583.
Now we perform the fourth step of the algorithm: p1 = 0.001 < 0.1 x (1/5), p2 = 0.012 < 0.1 x (2/5), p3 = 0.123 > 0.1 x (3/5), p4 = 0.473 > 0.1 x (4/5) and p5 = 0.583 > 0.1 x (5/5).
Only p1 and p2 meet the condition, the latter being the one that has the maximum value of the two, 0.012. Result: If we use this value as the new statistical significance threshold (instead of 0.05), on average, 10% of the time we reject the null hypothesis we will incur a type 1 error, which is the same that saying that we will detect a false positive.
Although this value may seem high, keep in mind that it is usually used in exploratory studies, such as the association with thousands of genes, which are used to open new theories of research. The findings will have to be confirmed.
To finish, I want you to notice a difference between the two adjustment methods that we have discussed. In the Bonferroni method, the new value of p does not depend on the data, but only on the number of contrasts that we want to perform, so we can know the adjusted value of p in advance.
However, this is not possible with the Benjamini-Hochberg method, since the new p value will depend on the data and the p values of the different contrasts. This is something similar to what happens with the Holm method, another adjustment method for multiple comparisons that is used under conditions similar to those of the Bonferroni method.
We are leaving…
And here we are going to leave it for today.
We have seen how chance constantly accompanies us in all our experiments and how it seems like a golden rule that we always have to give up something in order to improve something else. A little like life itself.
It has become clear to us how the risk of making a type 1 error and detecting a false positive increases with the number of comparisons we make, which is why it is necessary to apply some adjustment method.
When there are few comparisons, we can resort to adjusting the p value using methods such as Bonferroni, with which we will keep false positives at bay, doing little harm to true positives.
But when there are many comparisons, these methods can prevent us from detecting real effects, so it is necessary to change the type of approach and resign ourselves to having a certain proportion of false positives in order to continue detecting the true ones. We have looked at the Benjamini-Hochberg method to adjust our false discovery rate, although it is not the only possible method. For the virtuoso, there are several methods that use resampling techniques to calculate p and adjust the FDR. But that is another story…