Bayes’ factor
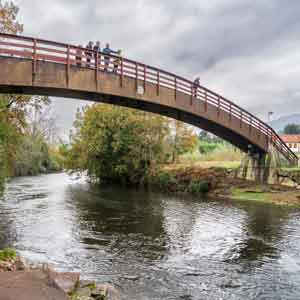
The concepts of odds and probability are described, as well as their combined use together with the Bayes’ factor for calculating the probability of being sick or healthy after knowing the result of a diagnostic test.
And I shoot because the current takes me. Surely you all know the game of the goose. It is a board game that already existed at the end of the 19th century, to entertain children and grown-ups.
It consists of a series of 63 squares, arranged in a spiral shape, which you have to go through from the beginning to the end, advancing a number of squares according to the score obtained by rolling one or two dice.
To liven up the game, there are some special squares that make you advance faster or move back part of what you have already advanced. Between these are the two squares with a bridge. If you fall into one of them, you move forward or backward to the other, while chanting “from bridge to bridge and I shoot because the current carries me”.
Thinking about going from one bridge to another, it has come to mind the back and forth that we can do in the field of probabilities between two widely used concepts, similar but different, such as the concepts of probability and odds.
So, although it is much less fun than the game of goose, today we are going to talk about probabilities and odds, how to go from one to the other as if we were going from bridge to bridge, and their applicability to calculating the probability of being sick after doing a diagnostic test.
You do not see the relationship between all this. Keep reading and you will understand.
Some preliminary definitions
In the first place, it is convenient to differentiate clearly between probability and odds .
Probability is the chance that a random event will occur. It is better to give an example than to try to explain it.
Imagine that we have a population of 100 people, 20 of whom suffer from that terrible disease that is fildulastrosis . We can calculate the probability of having the disease in that population. In other words, the probability that, if we choose an individual at random, this individual has fildulastrosis .
To do this, following the classic frequentist approach , we divided the number of favorable events (20 patients) by the total number of possible events (100 people): P = 20 / 100 = 0.2 = 20%. We can conclude, in this way, that the probability of suffering from fildulastrosis in our population is 20%.
As can be seen, probability is a proportion. It is important to understand that the proportion is a quotient in which the numerator is included in the denominator: the 20 patients in the numerator are included among the 100 individuals in the denominator.
An odds , which has its origins in games of chance, is a slightly different thing. In this case, the odds is a ratio between the individuals who have the characteristic and those who do not. In our example, it would be calculated as 20 sick / 80 healthy = 0.25.
But make no mistake, this is not a percentage, so we cannot say that the odds is 25%. The odds is not a proportion, because the numerator is not included in the denominator. Of course we could say that the odds of being sick to not being sick is 20:80 or, simplifying, 1:4. There will be one patient for every 4 healthy people in our population.
Thus, we can define the odds as the probability that an event occurs divided by the probability that it does not occur:
odds = P / (1 – P)
Odds, probability, and vice versa
We have already seen how to calculate the probability and the odds of an event to occur. In our example they are very similar but, as we saw in a previous post, if the frequency of the event we are studying is very high, the odds will tend to overestimate the association and will separate from the value of probability.
But what interests us this time is to see how we can go from one to another.
We have already seen the odds formula. Let us now see the probability formula expressed as a function of the odds of the event:
P = odds / (odds + 1)
Using these two formulas, we can easily go from one measurement to the other.
Seeing our example, the probability of having fildulastrosis is 0.2 (20%), so the odds will be 0.2 / (1 – 0.8) = 0.25.
And vice versa, if we know that the odds is 0.25, we can calculate that the probability will be 0.25 / (1 + 0.25) = 0.2 (20%).
And with this we have already seen the relationship between odds and probability. Let us now see how to apply all this to calculate the probability of being sick after carrying out a diagnostic test.
Bayes’ Factor
We already talked in a previous post about Bayes’ theorem, another child of games of chance. Well, from Bayes’ theorem, the Bayes’ factor (also known as Bayes’ rule) can be demonstrated, which says that the odds after a condition occurs is equal to the previous odds multiplied by a factor:
Posterior odds = Bayes’ factor x prior odds
And here comes the relationship of these concepts with the performance of diagnostic tests and the probability of being sick (or healthy) after having a positive (or negative) diagnostic test.
Let’s first see what the Bayes’ factor is. This is equivalent to the ratio of probabilities that an event will occur (or not) between those who have the event (sick) and those who do not (healthy). The most studious of you will have already realized that this is the likelihood ratio of a diagnostic test.
We can define the Bayes’ factor as follows:
Bayes’ factor = P(event|sick ) / P(event|not sick)
Let’s give an example to understand it better. In this case, the event will be having a positive fildulastrosis serology , knowing that this test has a sensitivity and specificity of 90% to classify fildulastrosis patients .
Bayes’ factor would be the probability of positive serology, fulfilling the fact of being sick. If we think about it a bit, this is the probability of testing positive when sick, which is nothing more than sensitivity.
On the other hand, the denominator of the Bayes’ factor would be the probability of having positive serology when healthy, which is nothing more than false positives, which we can define as the complement of specificity.
Bayes’ factor would be as follows:
Bayes’ Factor = S / (1 – Sp)
Now we see it clearly: in this case, the Bayes’ factor is the positive likelihood ratio.
Bayes’ factor is 0.9 / (1-0.9) = 9. That is, the positive likelihood ratio of serology as a diagnostic test is 9, which means that it is 9 times more likely that a sick person has a positive serology than a healthy one.
We can repeat this whole procedure considering what happens if the serology is negative. In this case:
Bayes factor = P(not happening|sick ) / P(not happening|not sick)
The probability of having negative serology (no event) in a patient is the percentage of false negatives, which can also be expressed as the complement of sensitivity (1-S). On the other hand, the probability of having it negative in a healthy person is nothing other than specificity. Thus, our Bayes’ factor , in this case, could be stated as
Bayes Factor = (1 – S) / Sp
Which, as we clearly see, is the negative likelihood ratio. In our example it would be (1 – 0.9) / 0.9 = 0.11. This means, approximately, that it is 9 times (1/0.11) more likely to have a negative serology in a healthy person than in a sick person.
From all this it can be concluded that a good diagnostic test will have a high positive likelihood ratio and a very low negative likelihood ratio.
From odds to probability, again
When we perform a diagnostic test on a patient, we want to know how likely is she to be sick based on the test result. However, the odds we have seen so far do not give us this information directly, although we can calculate it. Let’s see how.
What we usually know is the probability of being sick before taking the test, which is nothing more than the prevalence of the disease in the population from which the subject comes.
In our example population of 100 people we already know that the probability of having fildulastrosis is 0.2. We can already say that the prevalence or, what is the same, the pre-test probability , is 0.2.
From the pretest probability, we can calculate the pretest odds applying the formula that we already know: pre odds = 0.2/(1-0.2) = 0.25.
To calculate the posttest odds, we multiply the pretest odds by the Bayes’ factor. If we want to know the positive posttest odds, we multiply by the positive likelihood ratio: 0.25 x 9 = 2.25.
Finally, we apply the conversion formula that we already know to go from posttest odds to posttest probability: pos probability = 2.25/(1+2.25) = 0.69. This means that if we randomly choose a person from our sample population, do her a serology and it is positive, there is a 69% chance that she has the disease.
We can do the same for the negative result. We multiply the pretest odds by the negative likelihood ratio and we get the negative posttest odds: 0.25 x 0.11 = 0.02.
From this posttest odds, we calculate the negative posttest probability: 0.02/(1+0.02) = 0.02. This means that if the serology is negative, the probability of being sick is 2% (seen another way, the probability of being healthy is 98%).
Fagan’s nomogram
Everything we have seen so far is very cool. It allows us to calculate what we really want to know: the probability of being sick (or healthy) according to the result of the diagnostic test.
The problem, as you may have already noticed, is that the method is a bit cumbersome. It is for this reason that a graphical tool was designed that allows us to calculate the post-test probability, knowing the basal prevalence and the likelihood ratio, without mathematical calculations and in a simple way. This tool is the Fagan’s nomogram.
We are not going to comment now on how the Fagan’s nomogram is used so as not to lengthen this post too much, but I recommend that you read the previous post where we discussed it. You will see how simple and useful it can be to calculate the different post-test probabilities when the populations and their basal prevalence change.
We are leaving…
And that’s all for today.
We have seen the relationship between two similar but different concepts, such as odds and probability, and how to calculate either of them from the value of the other.
We have also seen the importance of the Bayes’ factor, which represents the likelihood ratios of the diagnostic test. Its great utility is that it allows us to calculate the post-test probability in populations with different prevalence of disease, virtue of which the positive and negative predictive values lack. But that is another story…